This tutorial will help explain which inference test should be used based upon the data set. Our discussion breaks down as follows:
1. Overview
Let's take a look at how to determine what type of hypothesis testing or inference test we should perform on a given data set. First, we need to ask ourselves if we're dealing with qualitative or quantitative data.
Type of Data
|
How Many Population Proportions or Population Means?
|
Test
|
Qualitative or Categorical Data
|
One Population Proportion
|
One-Proportion Z-Test; model the data using a normal distribution.
|
Two or More Population Proportions
|
Chi-squared test; determine if we are testing for goodness of fit, homogeneity, or association and independence.
|
|
Quantitative Data
|
One Population Mean
|
One-Sample Z-Test or a One-Sample T-Test; this will depend on whether or not we know the population standard deviation. If we do, we use the z-test. If we don't, we use the t-test.
|
Two Population Means
|
Special type of student t-test, which will not be addressed in this tutorial.
|
Three or More Population Means
|
ANOVA f-test; if our data has one characteristic, use a one-way ANOVA test. If it has two or more characteristics, use a two-way ANOVA test.
|
Another way to determine the type of test is through this inference test decision tree, which is available to view or download as a PDF at the end of this tutorial.
2. Qualitative or Categorical Data
2a. One-Proportion Z-Test
Suppose you hear that four out of five dentists recommend a certain type of toothpaste. After taking a sample of 100 dentists, you found that 75 dentists would recommend the toothpaste.
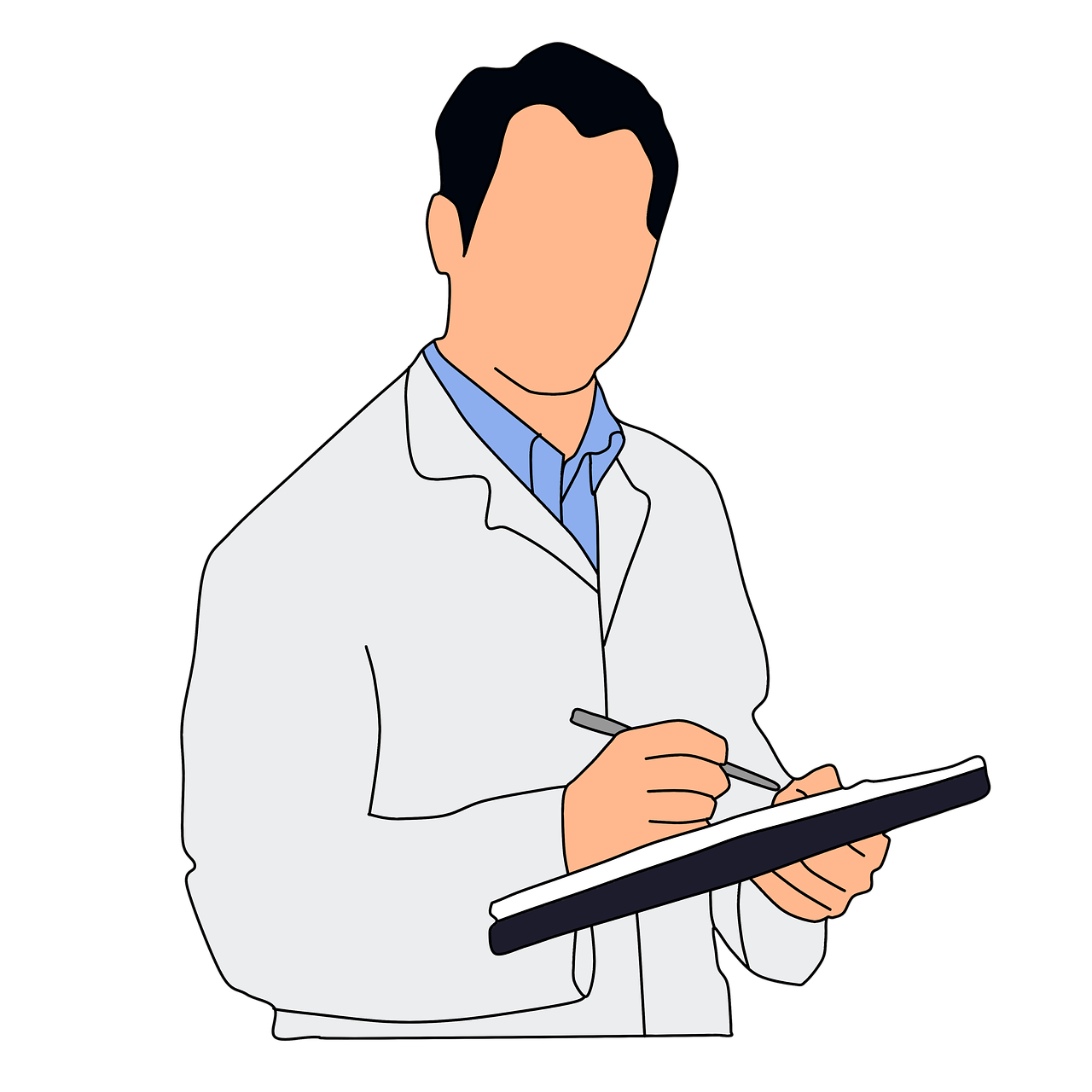 ✔
|
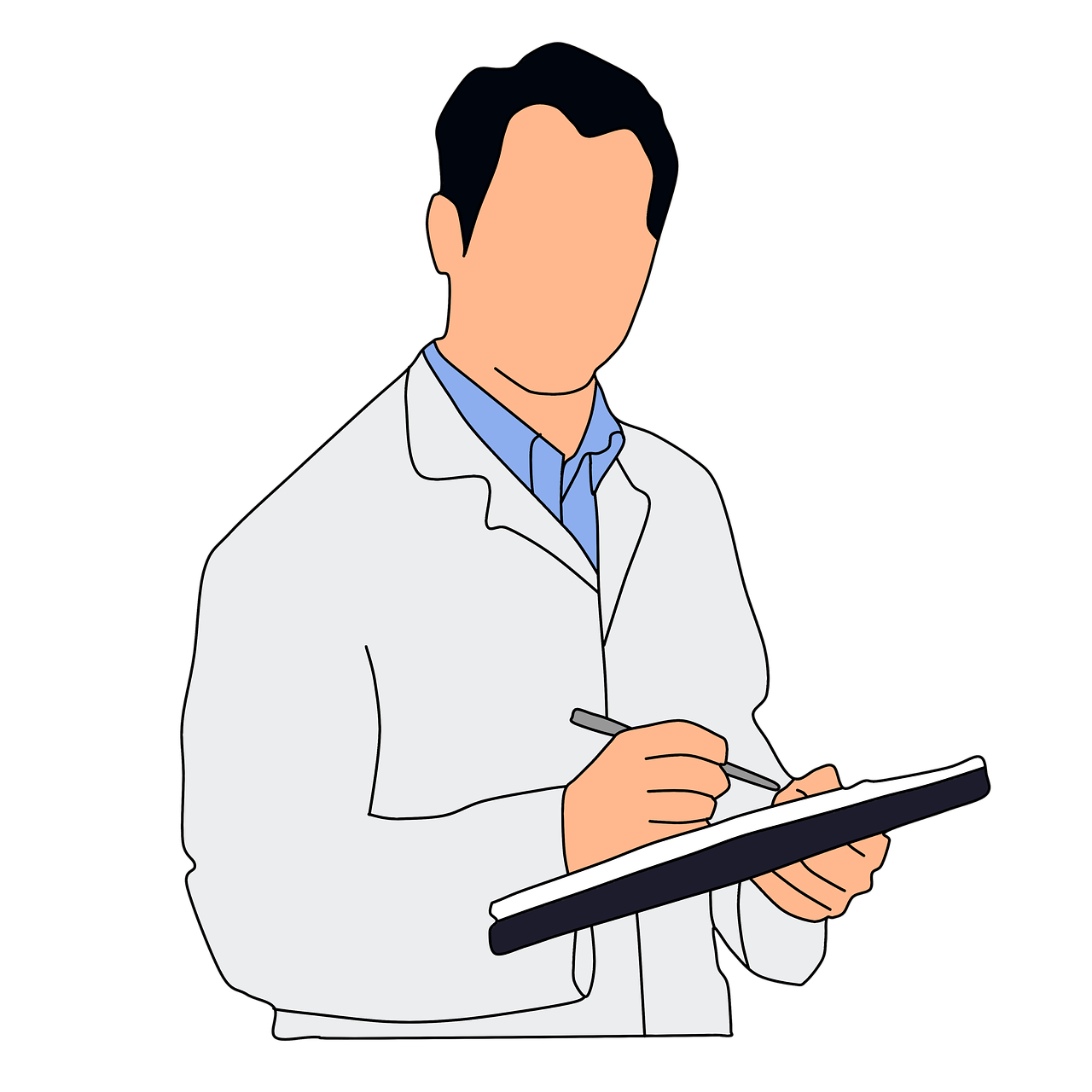 ✔
|
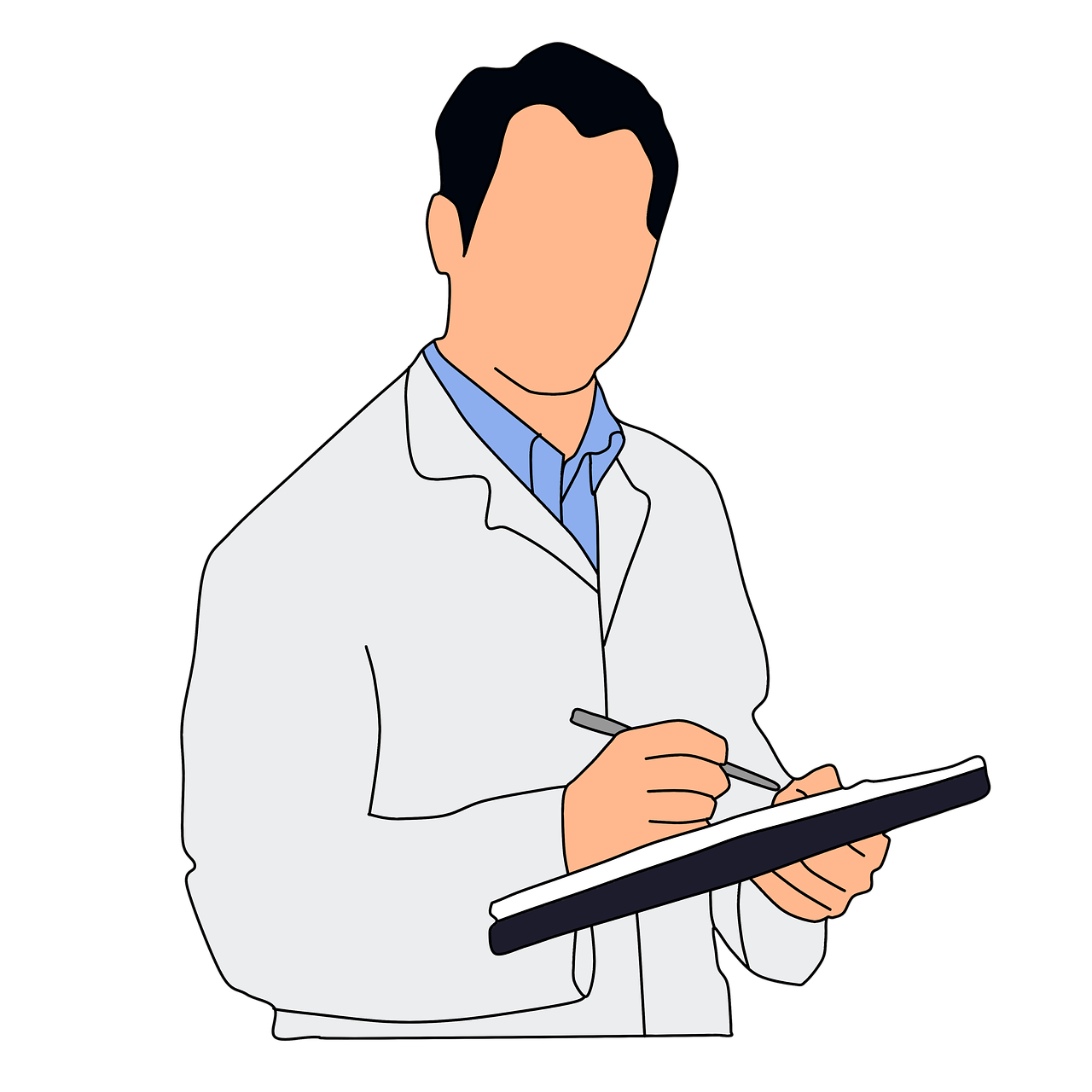 ✔
|
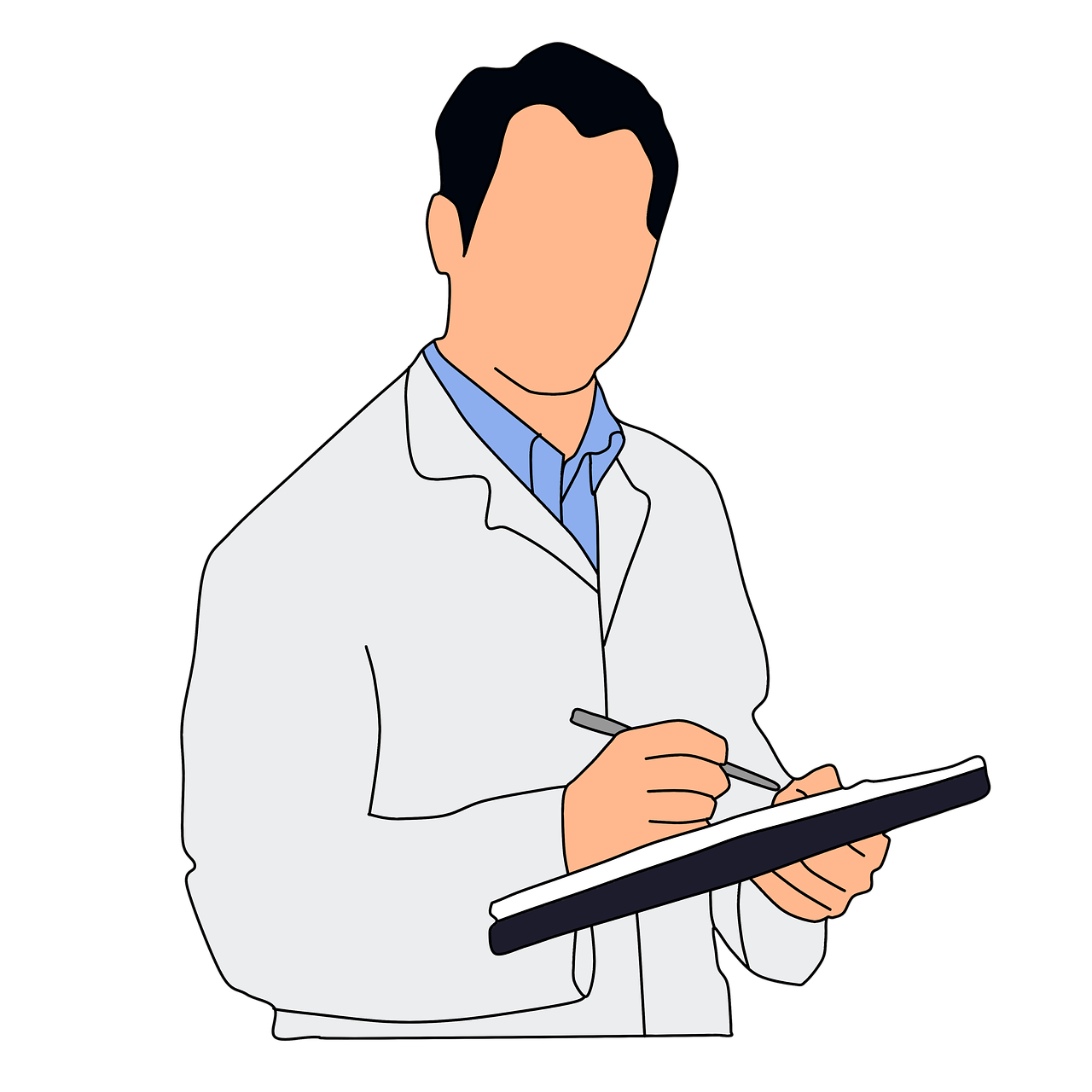 ✔
|
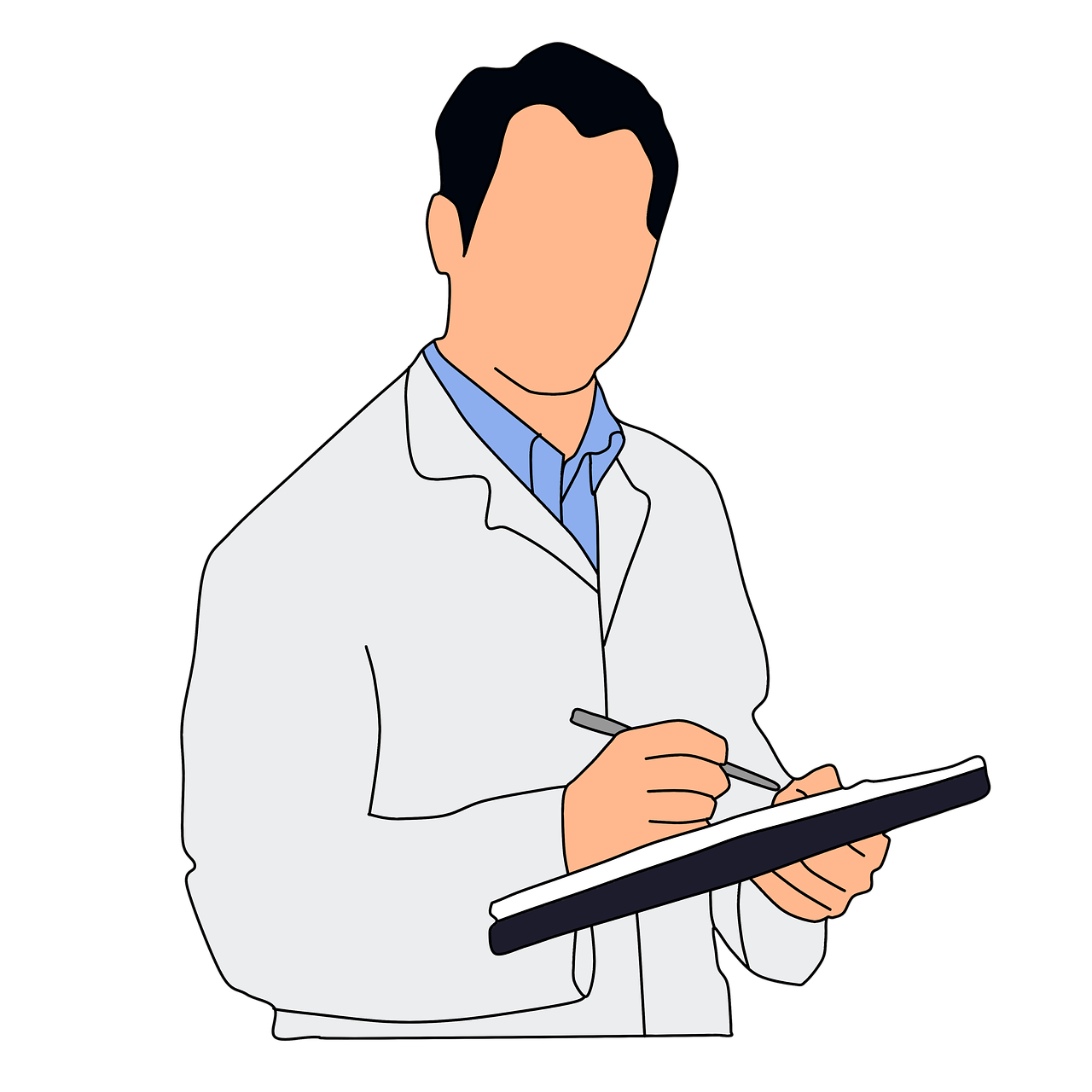 ✘
|
|
|
"Takes Away Cavities" Brand Toothpaste Sample Results
|
|
Was the claim accurate? What kind of tests are you going to use to try and figure this out?
- We need to note that we're dealing with categorical data here. We're looking at dentists and if they recommend something or don't recommend something. We're not really dealing with calculating means.
- We also need to think about how many proportions we have. Here we only have one proportion: 75 out of 100 dentists. Therefore, we're going to perform a one proportion z-test.
2b. Chi-Squared Test for Goodness-of-Fit
Suppose you flip a coin 100 times and recorded the number of heads and tails. In this case, we would expect that there would be 50 heads and 50 tails. However, our data showed 30 heads and 70 tails.
|
Heads
|
Tails
|
Expected
|
50
|
50
|
Observed
|
30
|
70
|
So, how can you tell if the coin that you're flipping is fair? And what tests should we use?
- We need to consider the type of data that we're dealing with. Notice here, we have heads and tails to record, which are categorical data because the data just falls into two categories: heads or tails.
- We're also dealing with population proportions in regards to heads and tails; there are two population proportions: heads and tails. Therefore, we're going to use a chi-squared test.
- But what kind of chi-squared test should we be using? We're comparing observed data to expected data. Because we are looking to see if the sample distribution matches the population distribution, we're going to be using a chi-squared test for goodness-of-fit.
2c. Chi-Squared Test for Homogeneity
Suppose you want to determine the effectiveness of the flu vaccine in preventing the chance of someone getting the flu. You gather data on 500 people where 250 had the flu vaccine, and 250 didn't get the flu vaccine. You also record who got the flu and who did not get it.
|
Caught Flu
|
Did Not Catch Flu
|
Total
|
Received Flu Vaccine
|
115
|
135
|
250
|
Did Not Receive Flu Vaccine
|
120
|
130
|
250
|
Received Flu Vaccine
|
235
|
265
|
500
|
What type of tests would you use to determine if the flu vaccine was effective or not?
- We need to ask ourselves again what kind of data we are dealing with. We're looking at those that got the flu vaccine and those who did not, as well as the number of people who caught the flu. Both are categorical data.
- Notice here that we're dealing with two populations proportions, those that got the flu and those who didn't. Therefore, we're going to use a chi-squared test again.
- We are also trying to determine if the flu vaccine was effective or not across two populations we're considering. Because we're seeing if there is a difference between this variable across two populations, we're going to use a chi-squared test for homogeneity.
2d. Chi-Squared Test for Association and Independence
Suppose we want to determine if gender affects whether or not someone likes an apple, orange, or banana.
How are we going to test this?
- We need to ask ourselves what kind of data we are dealing with. In this case, we're dealing with data that can be categorized by names--apples, oranges, and bananas, which are categorical data.
- We also notice that we're dealing with two populations proportions, men and women. Therefore, we're going to use a chi-squared test.
- We're trying to determine how apples, oranges, and bananas are related to each population. Because we are looking for an association between two or more variables in a single population, we're going to use a chi-squared test for association or independence.
3. Quantitative Data
3a. One-Way ANOVA
Suppose you're trying to determine if the overall standardized test scores on a given test across different states are equal for high school students trying to enter college.
What kind of test should we use?
- Notice that we are dealing with mean test scores here, which are qualitative data. Remember, that's the first thing you should always ask yourself. What kind of data am I dealing with?
- We're also dealing with several population means--in this case, 50 population means, one for each state. That means we're going to be using an ANOVA f-test.
- In this case, we're looking at one characteristic of the data, which is the overall test scores. Because we're just looking at one characteristic--overall test scores--we're going to use a one-way ANOVA f-test.
3b. Two-Way ANOVA
Suppose you want to determine how students in different states are performing on the Math and English sections of the exam.
How are we going to test this?
- We need to think about what kind of data we are dealing with. Here we're dealing with mean test scores, which are quantitative data.
- We're also dealing with multiple populations--in this case, up to 50 population means, because we're having one for each state. Again, because we have so many population means, we're going to use an ANOVA f-test.
- In this case, we're looking at two characteristics of the data tests: test scores on the math and the English section. So, we're going to use a two-way ANOVA f-test.
3c. One-Sample T-Test Vs. One-Sample Z-Test
Suppose we're concerned with the test scores of students in Minnesota taking a given standardized test.
How are we going to test this?
- Again, what kind of data are we dealing with? Here we're dealing with mean test scores, which are quantitative data.
- We're also dealing with one population mean--in this case, Minnesota's population mean. So, we're going to use a one-sample test.
- In this case, we're looking at one characteristic of the data, the overall test score. Therefore, if we don't know the standard deviation of the entire population that took the test, we would use a one-sample t-test. If, however, we did know the standard deviation of the population that took the test, then we would use a one-sample z-test.
This lesson explored how to perform different types of hypothesis or inference tests that you're likely to encounter when you're in a statistics course, and when to apply one over the other.